报告题目:Augmented Lagrangian method for image segmentation using elastica energy that prefers convex contours
报告时间:7月18日上午9:00-10:00
报告地点:基础教学部办公楼C08-201
报告人:台雪成教授
欢迎各位老师和研究生参加报告会!
报告摘要:
In the talk, we consider an Euler's elastica based image segmentation model. An interesting feature of this model lies in its preference of convex segmentation contour. However, due to the high order and non-differentiable term, it is often nontrivial to minimize the associated functional. In this work, we propose using augmented Lagrangian method to tackle the minimization problem. Especially, we design a novel augmented Lagrangian functional that deals with the mean curvature term differently as those ones in the previous works. The new treatment reduces the number of Lagrange multipliers employed, and more importantly, it helps represent the curvature more effectively and faithfully. Numerical experiments validate the efficiency of the proposed augmented Lagrangian method and also demonstrate new features of this particular segmentation model, such as shape driven and data driven properties.
报告人简介:
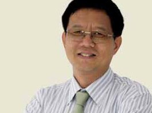
台雪成,挪威Bergen大学数学系教授,香港浸会大学数学系教授、新加坡南洋理工大学兼职教授,计算数学与科学工程计算、图像处理与分析领域国际知名学者,“冯康科学计算奖”获得者(2009年),新加坡“南洋杰出研究奖”(2010年)。台雪成教授首次提出并分析了具有快速和允许有稳定高效滤波性的加性算子分裂(AOS)算法,开创性地建立了分片常数水平集方法(PCLSM),开启了水平集思想处理图像的数学框架。在国际一流学术刊物及会议上发表论文逾百篇。